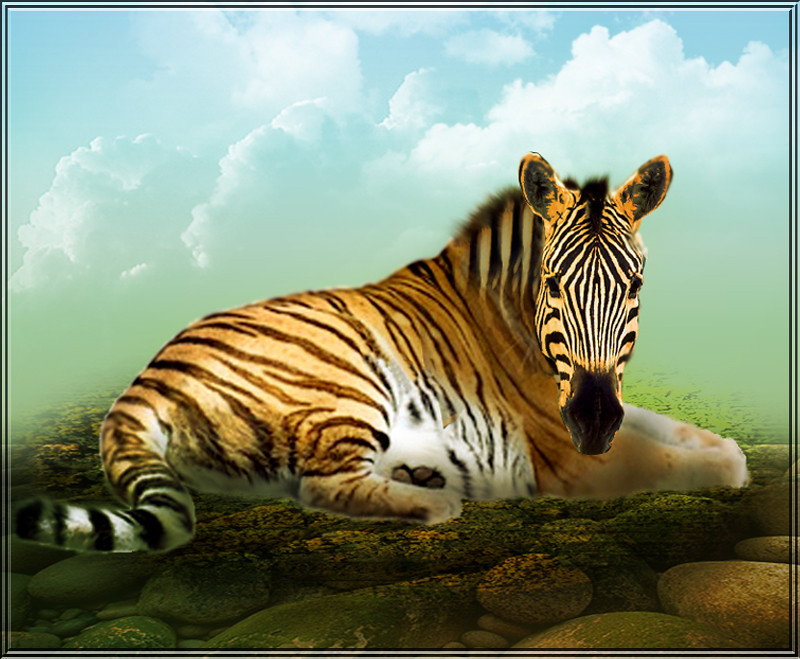
Most of the deep learning modeling is more or less black box modeling. There isn’t much one can do to inspect how an algorithm implements a particular task. A model can be biased by associating stripes with zebra more often than with a tiger. Human beings are biased. These prejudices and intuition helped them survive their journey through the wilderness.
Biases evolved along the way through different demographics, through cultures and other subconscious learnings. Computers, which are super rational, are expected to show dedication towards a job without any prejudice. But the data provided is generated by humans, curated by humans and then there is this ambitious pursuit towards AGI(human like intelligence). This is a walk on a tightrope for AI researchers who have to train the models for sophistications on human level while dodging the flaws like racism and other unwanted segregation.
An unbiased model is extremely crucial in large scale acceptance of AI in the coming years. There have been efforts to tackle bias through various means, by being cautious with data collection or curation. Google too proposes a novel approach to tackle bias, by testing with concept activation vectors(TCAV).
Testing With CAV
The concepts in concept activation(CAV) refers to a prediction class with color, gender or race; and other typical high level concepts used by humans to communicate. In traditional methods, the prediction is pivoted towards the weights given to features in an image which are low level like color intensity and other information retrieved by scanning hundreds of pixels.
The main objective of the researchers here is to provide ‘ high-dimensional internal state of a neural nets in human-friendly concepts’.
TCAV uses directional derivatives to quantify the model prediction’s sensitivity to a hidden high-level concept, learned by a concept activation vector.
Firstly a concept of interest is defined by choosing a set of examples that represent that concept. This allows the model to draw more insights other than the usual interpretations.
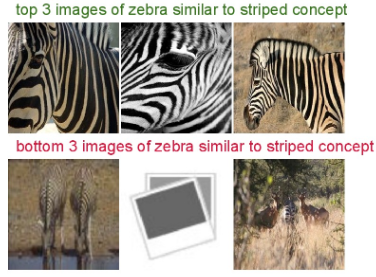
If a data scientist is tasked to train a model with some concept say striped objects, then a positive set having examples like tiger, zebra, lane crossing marks and a negative set containing random examples like pictures of ant, radio or pyramids are provided.
A collection of examples representing a certain concept, a vector is defined in the activations space. This vector is determined by considering the activations in the hidden layers from the input concepts and comparing them with random examples.
How Valid Is This Method
To test for the validation of CAVs, the researchers sorted the images of random class for inspection and then patterns of high activations are identified for visual confirmation.
As a CAV encodes the direction of a concept in the vector space, cosine similarity between a set of pictures is calculated. This helps in revealing any underlying biases as the sorting is done on similarities.
The experiments show that the concept ‘female’ is more relevant to the ‘apron’ class. Biases such as these can be inspected in the model and the quality of a certain concept can be determined. For example, the concept ‘apple’ should be shown more relevance to concepts like ‘red’ or ‘spherical’ and not ‘Newton’ or a ‘doctor’.
According to Google, TCAVs can be attributed with the following:
- TCAV requires little to no machine learning expertise from the user end.
- The range of concepts are not limited owing to TCAV’s adaptability.
- The machine learning model need not be modified to suit TCAV.
- Can draw insights from just one quantitative measure.
Know more about TCAV here