As we all know, Reinforcement Learning (RL) thrives on rewards and penalties but what if it is forced into situations where the environment doesn’t reward its actions?
A missing feedback component will render the model useless in sophisticated settings. But since reinforcement learning is exactly designed to do this, it is extremely important to investigate such shortcomings.
In what can be one of the most inventive strategies in the rich world of RL, a team of researchers from MIT, Princeton and DeepMind came up with something resourceful — to coordinate multiple agents by rewarding for the influence over other agents. This is a unique unified mechanism to encourage the agents to coordinate with each other in Multi-agent Reinforcement Learning (MARL).
Empathy Among Agents
An agent can be called as the unit cell of reinforcement learning. An agent receives rewards from the environment, it is optimised through algorithms to maximise this reward collection. And, complete the task. For example, when a robotic hand moves a chess piece or does a welding operation on automobiles, it is the agent, which drives the specific motors to move the arm.
But there can be scenarios where the reward system cannot be guaranteed. In this work, the researchers introduce a sublime way of teaching agents in reinforcement learning to behave for they will have some impact on other agents. In short, training the algorithm to be empathetic. This is similar to the accountability of actions in a social setting for humans. We speak and move in a certain way so as to influence or perhaps not to influence those in our vicinity- a case of causal inference.
The authors assess the causal influence in machines using counterfactual reasoning; at each time step, an agent simulates alternate, counterfactual actions that it could have taken, and assesses their effect on another agent’s behaviour. Actions that lead to a relatively higher change in the other agent’s behaviour are considered to be highly influential and are rewarded.
To study the influence reward, Sequential Social Dilemma (SSD) multi-agent environments was adopted.
Sequential Social Dilemmas (SSDs) are partially observable multi-agent games with a game-theoretic payoff structure where an individual agent can obtain a higher reward in the short-term by displaying non-cooperative behaviour but the total payoff per agent will be higher if all agents cooperate.
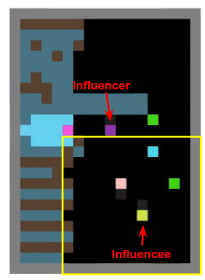
In one such game, apples (green tiles) provide the rewards but are a limited resource. Agents must coordinate harvesting apples with the behaviour of other agents in order to achieve cooperation. This method indicates how well the agents learned to cooperate.
The purple agent is trained with an intrinsic motivation to have a causal influence on other agents’ actions. It learns to use its actions as a kind of binary communication protocol, where it only uses ‘move right’ to traverse the map when apples are present and uses ‘turn left’ the rest of the time. Therefore, when another agent observes it moving right, it indicates the presence of apples which may be outside that agent’s field of view, allowing the purple agent to gain influence.
Unlike the other agents, which continue to move and explore randomly while waiting for apples to spawn, the influencer only traverses the map when it is pursuing an apple, then stops. The rest of the time it stays still.
Conclusion
The results of the experiments conducted in this study hint at the overall improvement of the model in the presence of intrinsic social influence. And, in some cases, it is clear that influence is essential to achieve any form of learning, attesting to the promise of this idea and highlighting the complexity of learning general deep neural network multi-agent policies.
To show that agents could use counterfactuals to develop a form of ‘empathy’, by simulating how their actions affect another agent’s value function is the first of its kind and this kind of influence could be used to drive coordinated behaviour in robots such as drones for surveillance/delivery or for pick and place robots at an assembly line.
Read the full work here.